! Disclaimer: IT experts, please hold on. This is a step-by-step guide for decision-makers to learn how and why to utilize retrieval augmented generation (RAG) and advanced technology to optimize management processes, digitalize and automate their business. Here you will find the general principles of retrieval augmented generation (RAG) systems, sprinkled with insights on the benefits, limitations and a unique use case bringing RAG and role-based access control together to adhere to your organizational governance. For those seeking more in-depth technical information, stay tuned for our next article starring Michael Weber, ValueMiner´s CTO.
Overview
- Why is retrieval augmented generation (RAG) important for your company?
- What is retrieval augmented generation (RAG)?
- What problem does RAG solve?
- How does RAG work? An example in 7 steps
- The benefits of RAG
- The limitations of retrieval augmented generation for businesses
- RAG use case: supercharge data security and compliance
Why is retrieval augmented generation (RAG) important for your company?
In our previous article titled Generative AI use cases to optimize your business processes, we mentioned one of the most critical limitations of large language models (LLM): AI hallucinations. LLM hallucinations happen especially when tools like ChatGPT, other cloud-based or in-house chatbots are asked about private and company-specific information.
The core of the problem is that cloud-based tools like ChatGPT are trained on publicly accessible data, excluding a company´s internal documents and industry-specific details. This gap can lead to inaccurate outputs, compromising the reliability that businesses need for handling sensitive operations and critical decision-making processes.
Retrieval augmented generation (RAG) minimizes AI Hallucinations and represents a significant leap forward in the application of AI for business, particularly for medium and large-sized companies.
What is retrieval augmented generation (RAG)?
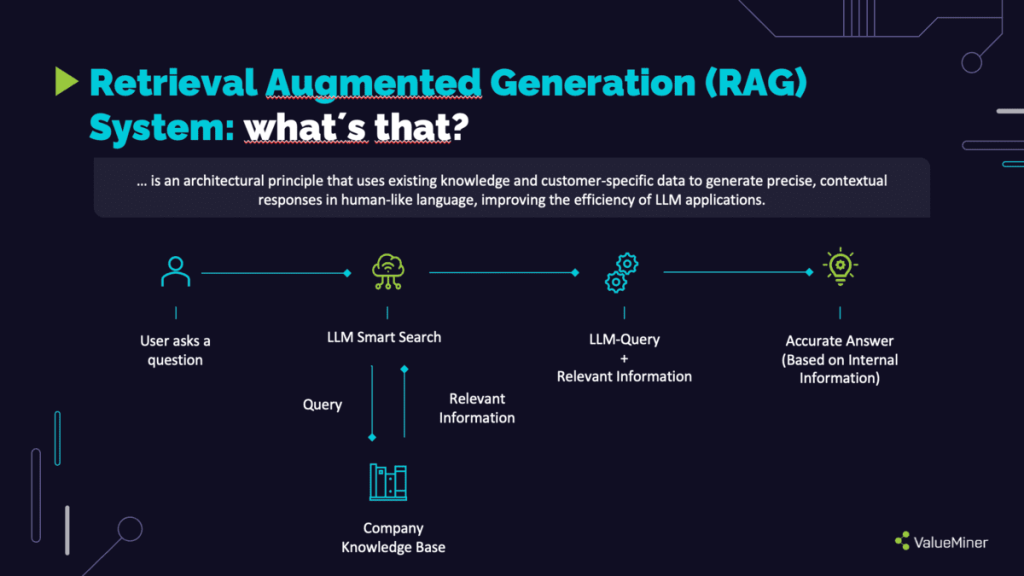
Retrieval augmented generation (RAG) is a system that amplifies the capabilities of large language models by using existing knowledge and customer-specific data to generate precise, contextual responses in human-like language.
At first glance, RAG may seem like a standard question and answer system – like ChatGPT – but under the hood is an impressively complex yet efficiently tuned system.
What problem does RAG solve?
In the age of AI, pre-trained large language models (LLMs) have emerged as a powerful tool for businesses, streamlining communication, optimizing management processes, automating tasks, and generating creative content.
However, most existing models are built on outdated and non-company-specific material, limiting their ability to generate knowledgeable answers that might contain company-based or domain-specific information.
The introduction of retrieval augmented generation (RAG) systems overcomes this challenge. The key strengths of RAG are its two mechanisms – retrieval and generation – which work together to combine information and produce smarter answers.
It means that RAG not only enables LLMs access and leverage your company´s valuable knowledge base – i.e. your data goldmine – transforming it into fuel for more informed, complete and relevant answers.
How does RAG work? An example in 7 steps
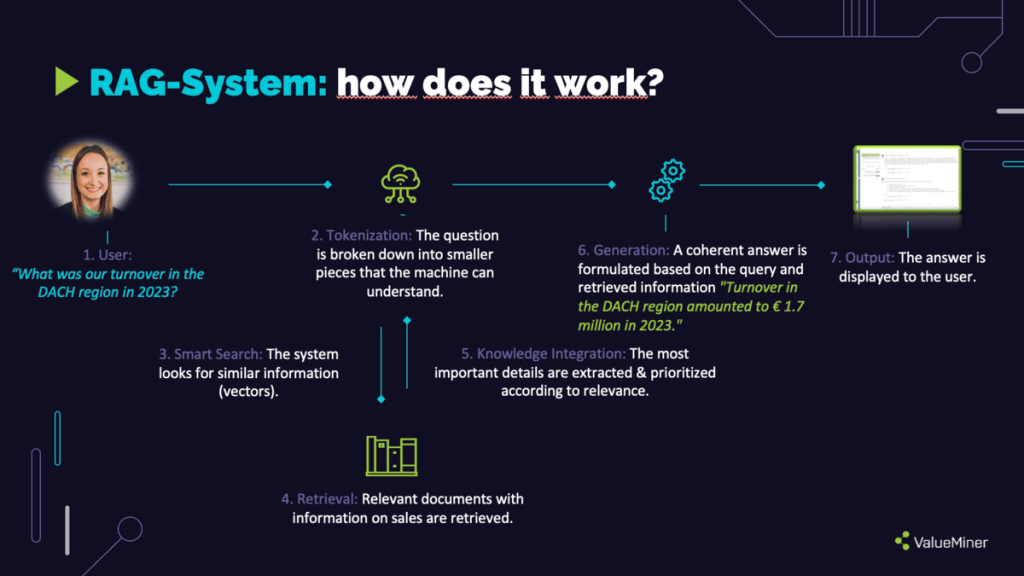
Step 1: Entering your query:
Start by asking your large language model (LLM) or your AI-chatbot a question.
Step 2: Processing information (Tokenization):
The system breaks down your query into smaller pieces (tokens), that the machine can understand. Think of it as a translating a book into a language that the computer can understand.
Step 3: Creating digital maps (Vectorization and Indexing):
Next, both query and stored information are converted into vectors.
Easy said, it means that they are converted into a kind of digital map. This helps the system compare information more effectively.
Step 4: Connecting and searching your company knowledge base (Retrieval)
The system connects to your company knowledge base through an application programming interface (API), which is like a bridge allowing the system to retrieve relevant documents.
When you ask a question, the system formulates a search request to retrieve the most relevant information or documents in your company knowledge base.
Step 5: Making sense of the result (Vectorization of Results)
The returned information is converted into the digital map format (vectors).
Step 6: Combining search and answering (combination of Retrieval and Generation)
The system combines the relevant information (vectors) with its generative capabilities to provide an accurate response to your initial query.
Step 7: Providing the answer to the user
Finally, the answer is displayed to the user.
In short, the retrieval augmented generation (RAG) system ensures that the generated content is both relevant and accurate, by leveraging a combination of retrieval from a specific knowledge base and generation capabilities using advanced AI models.
The benefits of RAG
- Interaction: With a RAG system you can delve deeply and easily through your company´s Know-How. Are you ready to chat with your own corporate knowledge data?
- Result: Precise results in real-time.
- Costs: Goodbye re-training – hello cost savings. Interestingly, while the process of training the generalized LLMs is time consuming and costly, updates to the retrieval augmented generation (RAG) system are just the opposite. New data can be loaded into the embedded language models and translated into vectors continuously. In fact, the responses of the entire generative AI system can be fed back into the RAG model. This improves its performance and accuracy because it knows how it has answered a similar question.
- Precision: Greater reliability in the outcomes.
- Privacy: RAG systems do not disclose sensitive data and minimize the risk of false information (aka, AI hallucinations).
- Transparency: Retrieval augmented generation systems provide the specific information source mentioned in its answers – something that LLMs cannot do.
The limitations of retrieval augmented generation (RAG) for businesses
When implementing a retrieval augmented generation system in your business, make sure to keep an eye on these sensitive topics:
- Complexity: Be aware that managing your own RAG infrastructure requires some maintenance, especially when it comes to updates and security checks. At the same time, make sure the system can work efficiently with larger amounts of data.
- Data quality: Even if RAG sounds impressive, a system is only as good as its data.
Watch out for inaccurate or outdated information included in your corporate knowledge base.
In fact, if the RAG model accesses inaccurate, or irrelevant data, this can lead to incorrect or misleading answers. Plan rigorous fact-check regularly to ensure the highest accuracy level!
- Data Protection: In general, we recommend avoiding unintentional internal disclosure of confidential or sensitive data.
On the one hand to external parties, like external cloud-based chatbot solutions, that are prone to security breaches. On the other hand, don´t forget that internal security matters too.
Protect sensitive information with the right access controls: not everyone needs access to everything in the company.
And this brings us to a very interesting topic…
RAG business use case: supercharge data security and compliance
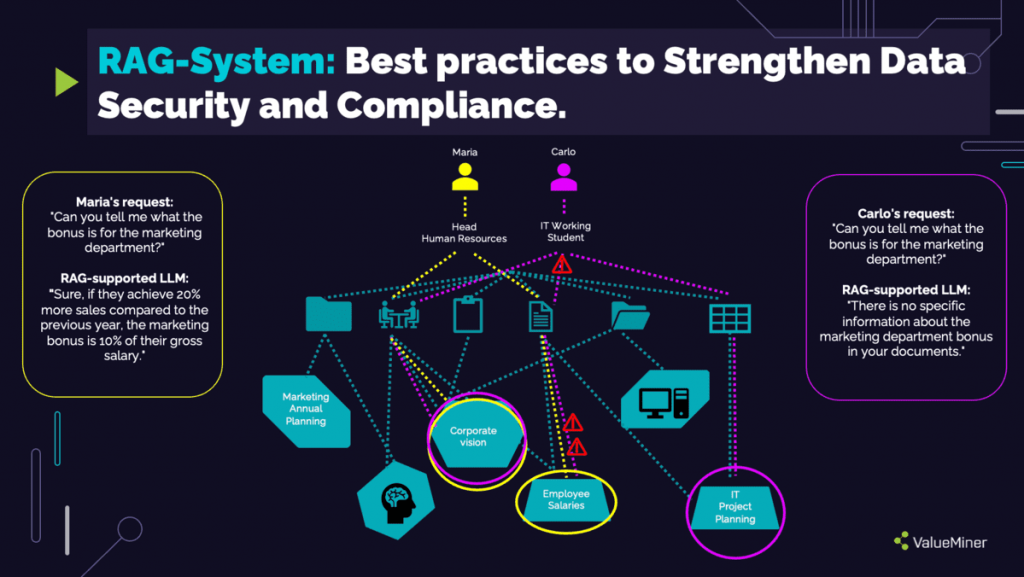
How can you make sure sensitive information remains protected, so that your smart AI assistant doesn’t tell everyone everything? Simply put, by restricting access to confidential information according to your company´s organizational structure and roles.
Most RAG systems often grant access either to single user documents or to all stored information, causing security risks and compliance issues.
To help our customers solve this urgent issue, we combined ValueMiner role-based data access control with RAG. It restricts information for AI assistants based on a user´s role within your company, enhancing governance compliance and operational efficiency.
With role-based access and retrieval augmented generation RAG, AI assistants deliver accurate information based on both business knowledge and user roles, ensuring only authorized employees see sensitive data, just like real-world business operations.
The benefits? Data stays secure, compliance is assured, and employees get the precise information they need.
Conclusions
Retrieval augmented generation (RAG) is a big step forward in the development of large language models. It empowers LLMs deliver clear and context-aware responses.
Leveraging RAG, you can finally transform your company knowledge into a strategic advantage.
To explore more, get in touch with our AI and business experts.